We consider the approximation of entropy solutions of nonlinear hyperbolic conservation laws using neural networks. We provide explicit computations that highlight why classical PINNs will not work for discontinuous solutions to nonlinear hyperbolic conservation laws and show that weak (dual) norms of the PDE residual should be used in the loss functional. This approach has been termed “weak PINNs” recently. We suggest some modifications to weak PINNs that make their training easier, which leads to smaller errors with less training, as shown by numerical experiments. Additionally, we extend wPINNs to scalar conservation laws with weak boundary data and to systems of hyperbolic conservation laws. We perform numerical experiments in order to assess the accuracy and efficiency of the extended method.
DOI: 10.5802/smai-jcm.116
Keywords: physics-informed learning, PINNs, hyperbolic conservation laws, entropy solution
Aidan Chaumet 1; Jan Giesselmann 1
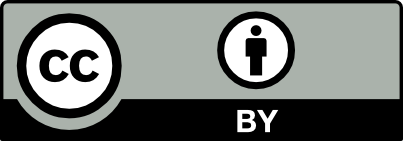
@article{SMAI-JCM_2024__10__373_0, author = {Aidan Chaumet and Jan Giesselmann}, title = {Improving {Weak} {PINNs} for {Hyperbolic} {Conservation} {Laws:} {Dual} {Norm} {Computation,} {Boundary} {Conditions} and {Systems}}, journal = {The SMAI Journal of computational mathematics}, pages = {373--401}, publisher = {Soci\'et\'e de Math\'ematiques Appliqu\'ees et Industrielles}, volume = {10}, year = {2024}, doi = {10.5802/smai-jcm.116}, zbl = {07963395}, language = {en}, url = {https://smai-jcm.centre-mersenne.org/articles/10.5802/smai-jcm.116/} }
TY - JOUR AU - Aidan Chaumet AU - Jan Giesselmann TI - Improving Weak PINNs for Hyperbolic Conservation Laws: Dual Norm Computation, Boundary Conditions and Systems JO - The SMAI Journal of computational mathematics PY - 2024 SP - 373 EP - 401 VL - 10 PB - Société de Mathématiques Appliquées et Industrielles UR - https://smai-jcm.centre-mersenne.org/articles/10.5802/smai-jcm.116/ DO - 10.5802/smai-jcm.116 LA - en ID - SMAI-JCM_2024__10__373_0 ER -
%0 Journal Article %A Aidan Chaumet %A Jan Giesselmann %T Improving Weak PINNs for Hyperbolic Conservation Laws: Dual Norm Computation, Boundary Conditions and Systems %J The SMAI Journal of computational mathematics %D 2024 %P 373-401 %V 10 %I Société de Mathématiques Appliquées et Industrielles %U https://smai-jcm.centre-mersenne.org/articles/10.5802/smai-jcm.116/ %R 10.5802/smai-jcm.116 %G en %F SMAI-JCM_2024__10__373_0
Aidan Chaumet; Jan Giesselmann. Improving Weak PINNs for Hyperbolic Conservation Laws: Dual Norm Computation, Boundary Conditions and Systems. The SMAI Journal of computational mathematics, Volume 10 (2024), pp. 373-401. doi : 10.5802/smai-jcm.116. https://smai-jcm.centre-mersenne.org/articles/10.5802/smai-jcm.116/
[1] First order quasilinear equations with boundary conditions, Commun. Partial Differ. Equations, Volume 4 (1979) no. 9, pp. 1017-1034 | DOI | MR | Zbl
[2] Least-Squares Finite Element Methods, Applied Mathematical Sciences, Springer, 2009 https://books.google.de/books?id=5ze_xil-fnqc | MR
[3] Hyperbolic conservation laws in continuum physics, Grundlehren der Mathematischen Wissenschaften, 325, Springer, 2016, xxxviii+826 pages | DOI | MR
[4] Minimal entropy conditions for Burgers equation, Q. Appl. Math., Volume 62 (2004) no. 4, pp. 687-700 | DOI | MR | Zbl
[5] On the approximation of functions by tanh neural networks, Neural Netw., Volume 143 (2021), pp. 732-750 | DOI | Zbl
[6] Generic bounds on the approximation error for physics-informed (and) operator learning, Advances in Neural Information Processing Systems 35 (NeurIPS 2022), Curran Associates, Inc. (2022), pp. 10945-10958 https://proceedings.neurips.cc/paper_files/paper/2022/file/46f0114c06524debc60ef2a72769f7a9-paper-conference.pdf
[7] wPINNs: Weak Physics Informed Neural Networks for Approximating Entropy Solutions of Hyperbolic Conservation Laws, SIAM J. Numer. Anal., Volume 62 (2024) no. 2, pp. 811-841 | DOI | MR | Zbl
[8] PINNs for the Solution of the Hyperbolic Buckley-Leverett Problem with a Non-convex Flux Function (2021) | arXiv
[9] Variational and topological methods for Dirichlet problems with -Laplacian, Port. Math. (N.S.), Volume 58 (2001) no. 3, pp. 339-378 | MR
[10] Neural-network-based approximations for solving partial differential equations, Commun. Numer. Methods Eng., Volume 10 (1994) no. 3, pp. 195-201 | DOI | Zbl
[11] The Deep Ritz Method: A Deep Learning-Based Numerical Algorithm for Solving Variational Problems, Commun. Math. Stat., Volume 6 (2018) no. 1, pp. 1-12 | DOI | MR | Zbl
[12] Gradient-annihilated PINNs for solving Riemann problems: Application to relativistic hydrodynamics, Comput. Methods Appl. Mech. Eng., Volume 424 (2024), 116906 | DOI | MR | Zbl
[13] Statistical Solutions of Hyperbolic Conservation Laws: Foundations, Arch. Ration. Mech. Anal., Volume 226 (2017) no. 2, pp. 809-849 | DOI | MR | Zbl
[14] Physics Informed Deep Learning for Flow and Transport in Porous Media, SPE Reservoir Simulation Conference (2021) | DOI
[15] The unreasonable effectiveness of adam on cycles, 33rd Conference on Neural Information Processing Systems (NeurIPS 2019), Vancouver, Canada (2019) https://sgo-workshop.github.io/cameraready2019/11.pdf
[16] A Finite Element Technique for Solving First-Order PDEs in , SIAM J. Numer. Anal., Volume 42 (2004) no. 2, pp. 714-737 | DOI | MR | Zbl
[17] A fast algorithm for solving first-order PDEs by -minimization, Commun. Math. Sci., Volume 6 (2008) no. 1, pp. 199-216 | DOI | MR | Zbl
[18] -minimization methods for Hamilton–Jacobi equations: the one-dimensional case, Numer. Math., Volume 109 (2008) no. 2, pp. 269-284 | DOI | MR | Zbl
[19] When Do Extended Physics-Informed Neural Networks (XPINNs) Improve Generalization?, SIAM J. Sci. Comput., Volume 44 (2022) no. 5, p. A3158-A3182 | DOI | MR | Zbl
[20] A Comparison Study of Deep Galerkin Method and Deep Ritz Method for Elliptic Problems with Different Boundary Conditions, Commun. Math. Res., Volume 36 (2020) no. 3, pp. 354-376 | DOI | MR | Zbl
[21] PyClaw: Accessible, Extensible, Scalable Tools for Wave Propagation Problems, SIAM J. Sci. Comput., Volume 34 (2012) no. 4, p. C210-C231 | DOI | MR | Zbl
[22] Variational Physics-Informed Neural Networks For Solving Partial Differential Equations (2019) | arXiv
[23] Adam: A Method for Stochastic Optimization, 3rd International Conference on Learning Representations, ICLR 2015, San Diego, CA, USA, May 7-9, 2015, Conference Track Proceedings (Yoshua Bengio; Yann LeCun, eds.) (2015) | arXiv
[24] Measure-valued solutions and well-posedness of multi-dimensional conservation laws in a bounded domain, Port. Math. (N.S.), Volume 58 (2001) no. 2, pp. 171-193 | MR | Zbl
[25] Artificial neural networks for solving ordinary and partial differential equations, IEEE Trans. Neural Netw., Volume 9 (1998) no. 5, pp. 987-1000 | DOI
[26] Neural-network methods for boundary value problems with irregular boundaries, IEEE Trans. Neural Netw., Volume 11 (2000) no. 5, pp. 1041-1049 | DOI
[27] Nonoscillatory solution of the steady-state inviscid burgers’ equation by mathematical programming, J. Comput. Phys., Volume 79 (1988) no. 2, pp. 436-448 | DOI | MR | Zbl
[28] Deep Nitsche Method: Deep Ritz Method with Essential Boundary Conditions, Commun. Comput. Phys., Volume 29 (2021) no. 5, pp. 1365-1384 | DOI | MR | Zbl
[29] Discontinuity Computing with Physics-Informed Neural Network, J. Sci. Comput., Volume 98 (2024) no. 1, 22 | DOI | MR | Zbl
[30] Non-diffusive neural network method for hyperbolic conservation laws, J. Comput. Phys., Volume 513 (2024), 113161 | DOI | MR | Zbl
[31] Deep learning observables in computational fluid dynamics, J. Comput. Phys., Volume 410 (2020), 109339 | DOI | MR | Zbl
[32] A priori and a posteriori error estimates for the Deep Ritz method applied to the Laplace and Stokes problem, J. Comput. Appl. Math., Volume 421 (2023), 114845 | DOI | Zbl
[33] Estimates on the generalization error of physics-informed neural networks for approximating PDEs, IMA J. Numer. Anal., Volume 43 (2022) no. 1, pp. 1-43 | DOI | MR
[34] fPINNs: Fractional Physics-Informed Neural Networks, SIAM J. Sci. Comput., Volume 41 (2019) no. 4, p. A2603-A2626 | DOI | MR | Zbl
[35] Uniqueness of the solution of the Cauchy problem for a first order quasilinear equation with one admissible strictly convex entropy, Math. Notes, Volume 55 (1994) no. 5, pp. 517-525 | DOI | Zbl
[36] Thermodynamically consistent physics-informed neural networks for hyperbolic systems, J. Comput. Phys., Volume 449 (2022), 110754 | DOI | MR | Zbl
[37] Physics-informed neural networks: A deep learning framework for solving forward and inverse problems involving nonlinear partial differential equations, J. Comput. Phys., Volume 378 (2019), pp. 686-707 | DOI | MR
[38] On the Convergence of Adam and Beyond, 6th International Conference on Learning Representations, ICLR 2018, Vancouver, BC, Canada, April 30 - May 3, 2018, Conference Track Proceedings (2018) https://openreview.net/forum?id=ryqu7f-rz
[39] Error Estimates of Residual Minimization using Neural Networks for Linear PDEs, J. Mach. Learn. Model. Comput., Volume 4 (2023) no. 4, pp. 73-101 | DOI
[40] DGM: A deep learning algorithm for solving partial differential equations, J. Comput. Phys., Volume 375 (2018), pp. 1339-1364 | DOI | MR | Zbl
[41] Cyclical Learning Rates for Training Neural Networks, 2017 IEEE Winter Conference on Applications of Computer Vision (WACV) (2017), pp. 464-472 | DOI
[42] A survey of several finite difference methods for systems of nonlinear hyperbolic conservation laws, J. Comput. Phys., Volume 27 (1978) no. 1, pp. 1-31 | DOI | MR | Zbl
[43] Physics informed neural networks: A case study for gas transport problems, J. Comput. Phys., Volume 481 (2023), 112041 | DOI | MR | Zbl
[44] Entropy solutions of the compressible Euler equations, BIT Numer. Math., Volume 56 (2016) no. 4, pp. 1479-1496 | DOI | MR | Zbl
[45] Is Physics Informed Loss Always Suitable for Training Physics Informed Neural Network?, Adv. Neural Inf. Process. Syst., Volume 35 (2022), pp. 8278-8290
[46] Respecting causality for training physics-informed neural networks, Comput. Methods Appl. Mech. Eng., Volume 421 (2024), 116813 | DOI | MR | Zbl
[47] Understanding and Mitigating Gradient Flow Pathologies in Physics-Informed Neural Networks, SIAM J. Sci. Comput., Volume 43 (2021) no. 5, p. A3055-A3081 | DOI | MR | Zbl
[48] When and why PINNs fail to train: A neural tangent kernel perspective, J. Comput. Phys., Volume 449 (2022), 110768 | DOI | MR | Zbl
Cited by Sources: