We consider the problem of approximating a function from $L^2$ by an element of a given $m$-dimensional space $V_m$, associated with some feature map $\varphi $, using evaluations of the function at random points $x_1, \dots ,x_n$. After recalling some results on optimal weighted least-squares using independent and identically distributed points, we consider weighted least-squares using projection determinantal point processes (DPP) or volume sampling. These distributions introduce dependence between the points that promotes diversity in the selected features $\varphi (x_i)$. We first provide a generalized version of volume-rescaled sampling yielding quasi-optimality results in expectation with a number of samples $n = O(m\log (m))$, that means that the expected $L^2$ error is bounded by a constant times the best approximation error in $L^2$. Also, further assuming that the function is in some normed vector space $H$ continuously embedded in $L^2$, we further prove that the approximation error in $L^2$ is almost surely bounded by the best approximation error measured in the $H$-norm. This includes the cases of functions from $L^\infty $ or reproducing kernel Hilbert spaces. Finally, we present an alternative strategy consisting in using independent repetitions of projection DPP (or volume sampling), yielding similar error bounds as with i.i.d. or volume sampling, but in practice with a much lower number of samples. Numerical experiments illustrate the performance of the different strategies.
Anthony Nouy 1; Bertrand Michel 1
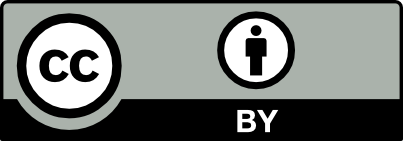
@article{SMAI-JCM_2025__11__1_0, author = {Anthony Nouy and Bertrand Michel}, title = {Weighted least-squares approximation with determinantal point processes and generalized volume sampling}, journal = {The SMAI Journal of computational mathematics}, pages = {1--36}, publisher = {Soci\'et\'e de Math\'ematiques Appliqu\'ees et Industrielles}, volume = {11}, year = {2025}, doi = {10.5802/smai-jcm.117}, language = {en}, url = {https://smai-jcm.centre-mersenne.org/articles/10.5802/smai-jcm.117/} }
TY - JOUR AU - Anthony Nouy AU - Bertrand Michel TI - Weighted least-squares approximation with determinantal point processes and generalized volume sampling JO - The SMAI Journal of computational mathematics PY - 2025 SP - 1 EP - 36 VL - 11 PB - Société de Mathématiques Appliquées et Industrielles UR - https://smai-jcm.centre-mersenne.org/articles/10.5802/smai-jcm.117/ DO - 10.5802/smai-jcm.117 LA - en ID - SMAI-JCM_2025__11__1_0 ER -
%0 Journal Article %A Anthony Nouy %A Bertrand Michel %T Weighted least-squares approximation with determinantal point processes and generalized volume sampling %J The SMAI Journal of computational mathematics %D 2025 %P 1-36 %V 11 %I Société de Mathématiques Appliquées et Industrielles %U https://smai-jcm.centre-mersenne.org/articles/10.5802/smai-jcm.117/ %R 10.5802/smai-jcm.117 %G en %F SMAI-JCM_2025__11__1_0
Anthony Nouy; Bertrand Michel. Weighted least-squares approximation with determinantal point processes and generalized volume sampling. The SMAI Journal of computational mathematics, Volume 11 (2025), pp. 1-36. doi : 10.5802/smai-jcm.117. https://smai-jcm.centre-mersenne.org/articles/10.5802/smai-jcm.117/
[1] Faster subset selection for matrices and applications, SIAM J. Matrix Anal. Appl., Volume 34 (2013) no. 4, pp. 1464-1499 | DOI | MR | Zbl
[2] Constructive subsampling of finite frames with applications in optimal function recovery, Appl. Comput. Harmon. Anal., Volume 65 (2023), pp. 209-248 | DOI | MR | Zbl
[3] A Faster Sampler for Discrete Determinantal Point Processes, Proceedings of the 26th International Conference on Artificial Intelligence and Statistics (Proceedings of Machine Learning Research), Volume 206, PMLR, 2023, pp. 5582-5592 https://proceedings.mlr.press/v206/barthelme23a.html
[4] Kernel interpolation with continuous volume sampling, Proceedings of the 37th International Conference on Machine Learning (Proceedings of Machine Learning Research), Volume 119, PMLR, 2020, pp. 725-735 https://proceedings.mlr.press/v119/belhadji20a.html
[5] Signal reconstruction using determinantal sampling (2023) | arXiv
[6] Reproducing kernel Hilbert spaces in probability and statistics, Springer, 2011 | MR
[7] Convergence rates for greedy algorithms in reduced basis methods, SIAM J. Math. Anal., Volume 43 (2011) no. 3, pp. 1457-1472 | DOI | MR | Zbl
[8] Computing Multivariate Fekete and Leja Points by Numerical Linear Algebra, SIAM J. Numer. Anal., Volume 48 (2010) no. 5, pp. 1984-1999 | DOI | MR | Zbl
[9] Optimal weighted least-squares methods, SMAI J. Comput. Math., Volume 3 (2017), pp. 181-203 | DOI | Numdam | MR | Zbl
[10] Unbiased estimators for random design regression, J. Mach. Learn. Theory, Volume 23 (2022) no. 1, pp. 7539-7584
[11] Matrix approximation and projective clustering via volume sampling, Theory Comput., Volume 2 (2006) no. 1, pp. 225-247 | DOI | MR
[12] Randomized Least-Squares with Minimal Oversampling and Interpolation in General Spaces, SIAM J. Numer. Anal., Volume 62 (2024) no. 4, pp. 1515-1538 | DOI | MR
[13] Optimal pointwise sampling for approximation, J. Complexity, Volume 68 (2022), 101602, 12 pages | MR
[14] A sharp upper bound for sampling numbers in , Appl. Comput. Harmon. Anal., Volume 63 (2023), pp. 113-134 | DOI | MR
[15] Efficient rectangular maximal-volume algorithm for rating elicitation in collaborative filtering, 2016 IEEE 16th International Conference on Data Mining (ICDM), IEEE (2016), pp. 141-150 | DOI
[16] The maximal-volume concept in approximation by low-rank matrices, Structured matrices in mathematics, computer science, and engineering I (Contemporary Mathematics), Volume 280, American Mathematical Society, 2001, pp. 47-52 | Zbl
[17] Boosted optimal weighted least-squares, Math. Comput., Volume 91 (2022) no. 335, pp. 1281-1315 | MR | Zbl
[18] Kernel-based interpolation at approximate Fekete points, Numer. Algorithms, Volume 87 (2021) no. 1, pp. 445-468 | DOI | MR | Zbl
[19] Sampling discretization and related problems, J. Complexity, Volume 71 (2022), 101653, 55 pages | DOI | MR | Zbl
[20] Sampling projections in the uniform norm (2024) | arXiv
[21] Determinantal point process models and statistical inference, J. R. Stat. Soc., Ser. B, Stat. Methodol., Volume 77 (2015) no. 4, pp. 853-877 | DOI | MR | Zbl
[22] A general multipurpose interpolation procedure: the magic points, Commun. Pure Appl. Anal., Volume 8 (2008), pp. 383-404 | DOI | MR | Zbl
[23] Interlacing families II: Mixed characteristic polynomials and the Kadison–Singer problem, Ann. Math., Volume 182 (2015) no. 1, pp. 327-350 | DOI | Zbl
[24] Exponential frames on unbounded sets, Proc. Am. Math. Soc., Volume 144 (2016) no. 1, pp. 109-118 | DOI | MR | Zbl
[25] On proportional volume sampling for experimental design in general spaces, Stat. Comput., Volume 33 (2022) no. 1, 29, 22 pages | DOI | MR | Zbl
[26] A Note on Sampling Recovery of Multivariate Functions in the Uniform Norm, SIAM J. Numer. Anal., Volume 60 (2022) no. 3, pp. 1363-1384 | DOI | MR
[27] Optimal design of experiments, Classics in Applied Mathematics, 50, Society for Industrial and Applied Mathematics, 2006, xxix+454 pages | DOI | MR | Zbl
[28] On the power of iid information for linear approximation (2023) | arXiv
[29] User-friendly tail bounds for sums of random matrices, Found. Comput. Math., Volume 12 (2012) no. 4, pp. 389-434 | DOI | MR | Zbl
[30] An introduction to matrix concentration inequalities, Found. Trends Mach. Learn., Volume 8 (2015) no. 1-2, pp. 1-230 | DOI
[31] Almost-sure quasi-optimal approximation in reproducing kernel Hilbert spaces (2024) | arXiv
Cited by Sources: