Given samples of a function in random points drawn with respect to a measure we develop theoretical analysis of the -approximation error. For a parituclar choice of depending on , it is known that the weighted least squares method from finite dimensional function spaces , has the same error as the best approximation in up to a multiplicative constant when given exact samples with logarithmic oversampling. If the source measure and the target measure differ we are in the domain adaptation setting, a subfield of transfer learning. We model the resulting deterioration of the error in our bounds.
Further, for noisy samples, our bounds describe the bias-variance trade off depending on the dimension of the approximation space . All results hold with high probability.
For demonstration, we consider functions defined on the -dimensional cube given in unifom random samples. We analyze polynomials, the half-period cosine, and a bounded orthonormal basis of the non-periodic Sobolev space . Overcoming numerical issues of this basis, this gives a novel stable approximation method with quadratic error decay. Numerical experiments indicate the applicability of our results.
Keywords: domain adaptation, individual function approximation, least squares, sampling theory, transfer learning, unit cube, polynomial approximation
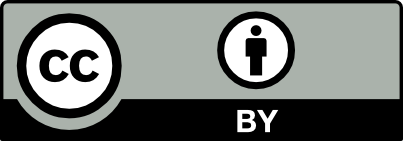
@article{SMAI-JCM_2023__9__95_0, author = {Felix Bartel}, title = {Error {Guarantees} for {Least} {Squares} {Approximation} with {Noisy} {Samples} in {Domain} {Adaptation}}, journal = {The SMAI Journal of computational mathematics}, pages = {95--120}, publisher = {Soci\'et\'e de Math\'ematiques Appliqu\'ees et Industrielles}, volume = {9}, year = {2023}, doi = {10.5802/smai-jcm.96}, language = {en}, url = {https://smai-jcm.centre-mersenne.org/articles/10.5802/smai-jcm.96/} }
TY - JOUR AU - Felix Bartel TI - Error Guarantees for Least Squares Approximation with Noisy Samples in Domain Adaptation JO - The SMAI Journal of computational mathematics PY - 2023 SP - 95 EP - 120 VL - 9 PB - Société de Mathématiques Appliquées et Industrielles UR - https://smai-jcm.centre-mersenne.org/articles/10.5802/smai-jcm.96/ DO - 10.5802/smai-jcm.96 LA - en ID - SMAI-JCM_2023__9__95_0 ER -
%0 Journal Article %A Felix Bartel %T Error Guarantees for Least Squares Approximation with Noisy Samples in Domain Adaptation %J The SMAI Journal of computational mathematics %D 2023 %P 95-120 %V 9 %I Société de Mathématiques Appliquées et Industrielles %U https://smai-jcm.centre-mersenne.org/articles/10.5802/smai-jcm.96/ %R 10.5802/smai-jcm.96 %G en %F SMAI-JCM_2023__9__95_0
Felix Bartel. Error Guarantees for Least Squares Approximation with Noisy Samples in Domain Adaptation. The SMAI Journal of computational mathematics, Volume 9 (2023), pp. 95-120. doi : 10.5802/smai-jcm.96. https://smai-jcm.centre-mersenne.org/articles/10.5802/smai-jcm.96/
[1] Multivariate modified Fourier series and application to boundary value problems, Numer. Math., Volume 115 (2010) no. 4, pp. 511-552 | DOI | MR | Zbl
[2] Multivariate modified Fourier expansions, Spectral and high order methods for partial differential equations (Lecture Notes in Computational Science and Engineering), Volume 76, Springer, 2011, pp. 85-92 | DOI | MR | Zbl
[3] From high oscillation to rapid approximation II: expansions in Birkhoff series, IMA J. Numer. Anal., Volume 32 (2012) no. 1, pp. 105-140 | DOI | MR | Zbl
[4] Model selection for regression on a random design, ESAIM, Probab. Stat., Volume 6 (2002), pp. 127-146 | DOI | Numdam | MR | Zbl
[5] Concentration inequalities for cross-validation in scattered data approximation, J. Approx. Theory, Volume 277 (2022), 105715, 17 pages | DOI | MR | Zbl
[6] Fast Cross-validation in Harmonic Approximation, Appl. Comput. Harmon. Anal., Volume 49 (2020) no. 2, pp. 415-437 | DOI | MR | Zbl
[7] Constructive subsampling of finite frames with applications in optimal function recovery, Appl. Comput. Harmon. Anal. (2023) (to appear) | DOI | MR | Zbl
[8] Concentration of quadratic forms under a Bernstein moment assumption (2019) (https://arxiv.org/abs/1901.08736)
[9] Discrete least squares polynomial approximation with random evaluations—application to parametric and stochastic elliptic PDEs, ESAIM, Math. Model. Numer. Anal., Volume 49 (2015) no. 3, pp. 815-837 | DOI | Numdam | MR | Zbl
[10] On the stability and accuracy of least squares approximations, Found. Comput. Math., Volume 13 (2013) no. 5, pp. 819-834 | DOI | MR | Zbl
[11] Optimal weighted least-squares methods, SMAI J. Comput. Math., Volume 3 (2017), pp. 181-203 | DOI | Numdam | MR | Zbl
[12] Tent-transformed lattice rules for integration and approximation of multivariate non-periodic functions, J. Complexity, Volume 36 (2016), pp. 166-181 | DOI | MR | Zbl
[13] Lattice rules for nonperiodic smooth integrands, Numer. Math., Volume 126 (2014) no. 2, pp. 259-291 | DOI | MR | Zbl
[14] Optimal pointwise sampling for approximation, J. Complexity, Volume 68 (2022), 101602 | DOI | MR | Zbl
[15] Optimal sampling and Christoffel functions on general domains, Constr. Approx., Volume 56 (2022) no. 1, pp. 121-163 | DOI | MR | Zbl
[16] A sharp upper bound for sampling numbers in , Appl. Comput. Harmon. Anal., Volume 63 (2023), pp. 113-134 | DOI | MR
[17] A mathematical introduction to compressive sensing, Applied and Numerical Harmonic Analysis, Birkhäuser/Springer, 2013, xviii+625 pages | DOI | MR
[18] On a regularization of unsupervised domain adaptation in RKHS, Appl. Comput. Harmon. Anal., Volume 57 (2022), pp. 201-227 | DOI | MR | Zbl
[19] Iterative methods for solving linear systems, Frontiers in Applied Mathematics, 17, Society for Industrial and Applied Mathematics, 1997, xiv+220 pages | DOI | MR
[20] A distribution-free theory of nonparametric regression, Springer Series in Statistics, Springer, 2002, xvi+647 pages | DOI | MR
[21] Boosted optimal weighted least-squares, Math. Comput. (2022) | DOI | MR | Zbl
[22] Coherence motivated sampling and convergence analysis of least squares polynomial Chaos regression, Comput. Methods Appl. Mech. Eng., Volume 290 (2015), pp. 73-97 | DOI | MR | Zbl
[23] From high oscillation to rapid approximation. I. Modified Fourier expansions, IMA J. Numer. Anal., Volume 28 (2008) no. 4, pp. 862-887 | DOI | MR | Zbl
[24] Worst-case recovery guarantees for least squares approximation using random samples, Constr. Approx., Volume 54 (2021) no. 2, pp. 295-352 | DOI | MR | Zbl
[25] On a special class of differential operators, Dokl. Akad. Nauk SSSR, Volume 2 (1935), pp. 345-349
[26] Function values are enough for -approximation, Found. Comput. Math., Volume 21 (2021) no. 4, pp. 1141-1151 | DOI | MR | Zbl
[27] Function integration, reconstruction and approximation using rank-1 lattices, Math. Comput., Volume 90 (2021) no. 330, pp. 1861-1897 | DOI | MR | Zbl
[28] On sampling discretization in , J. Math. Anal. Appl., Volume 515 (2022) no. 2, 126457, 14 pages | DOI | MR | Zbl
[29] Fast Hyperbolic Wavelet Regression meets ANOVA (2021) (https://arxiv.org/abs/2108.13197, to appear in Numer. Math.) | DOI
[30] Balancing principle in supervised learning for a general regularization scheme, Appl. Comput. Harmon. Anal., Volume 48 (2020) no. 1, pp. 123-148 | DOI | MR | Zbl
[31] Analysis of Discrete Projection on Polynomial Spaces with Random Evaluations, Found. Comput. Math. (2014) | DOI | MR | Zbl
[32] -norm sampling discretization and recovery of functions from RKHS with finite trace, Sampl. Theory Signal Process. Data Anal., Volume 19 (2021) no. 2, 13, 31 pages | DOI | MR | Zbl
[33] A New Upper Bound for Sampling Numbers, Found. Comput. Math. (2021) | DOI
[34] A Christoffel function weighted least squares algorithm for collocation approximations, Math. Comput., Volume 86 (2017) no. 306, pp. 1913-1947 | DOI | MR | Zbl
[35] A note on transformed Fourier systems for the approximation of non-periodic signals, Monte Carlo and quasi-Monte Carlo methods (Springer Proceedings in Mathematics & Statistics), Volume 387, Springer, 2022, pp. 253-271 | DOI | MR
[36] A Survey on Transfer Learning, IEEE Trans. Knowl. Data Eng., Volume 22 (2010) no. 10, pp. 1345-1359 | DOI
[37] Regularization Theory for Ill-Posed Problems. Selected Topics, Walter de Gruyter, 2013, 287 pages | DOI
[38] Numerical Fourier analysis, Applied and Numerical Harmonic Analysis, Birkhäuser/Springer, 2018, xvi+168 pages | DOI | MR
[39] Learning multivariate functions with low-dimensional structures using polynomial bases, J. Comput. Appl. Math., Volume 403 (2022), 113821, 19 pages | DOI | MR | Zbl
[40] Fast and exact reconstruction of arbitrary multivariate algebraic polynomials in Chebyshev form, 2015 International Conference on Sampling Theory and Applications (SampTA) (2015), pp. 392-396 | DOI
[41] A Note on Sampling Recovery of Multivariate Functions in the Uniform Norm, SIAM J. Numer. Anal., Volume 60 (2022) no. 3, pp. 1363-1384 | DOI | MR | Zbl
[42] Sparse Legendre expansions via -minimization, J. Approx. Theory, Volume 164 (2012) no. 5, pp. 517-533 | DOI | MR
[43] Hanson-Wright inequality and sub-gaussian concentration, Electron. Commun. Probab., Volume 18 (2013), 82, 9 pages | DOI | MR | Zbl
[44] Spectral methods. Algorithms, analysis and applications, Springer Series in Computational Mathematics, 41, Springer, 2011, xvi+470 pages | DOI | MR
[45] Support vector machines, Information Science and Statistics, Springer, 2008, xvi+601 pages | MR
[46] Reconstruction and collocation of a class of non-periodic functions by sampling along tent-transformed rank-1 lattices, J. Fourier Anal. Appl., Volume 22 (2016) no. 1, pp. 187-214 | DOI | MR | Zbl
[47] On approximate recovery of functions with bounded mixed derivative, J. Complexity, Volume 9 (1993) no. 1, pp. 41-59 (Festschrift for Joseph F. Traub, Part I) | DOI | MR | Zbl
[48] Approximation theory and approximation practice, Society for Industrial and Applied Mathematics, 2013, viii+305 pages | DOI | MR
[49] Theory of function spaces, Modern Birkhäuser Classics, Birkhäuser, 2010, 285 pages | MR
[50] Theory of function spaces. II, Monographs in Mathematics, 84, Birkhäuser, 1992, viii+370 pages | DOI | MR
[51] User-friendly tail bounds for sums of random matrices, Found. Comput. Math., Volume 12 (2012) no. 4, pp. 389-434 | DOI | MR | Zbl
[52] New error bounds for Legendre approximations of differentiable functions (2021) (https://arxiv.org/abs/2111.03833) | DOI
[53] Tractability of Multivariate Approximation over a Weighted Unanchored Sobolev Space, Constr. Approx., Volume 30 (2009) no. 3, pp. 395-421 | DOI | MR | Zbl
Cited by Sources: