We consider the problem of reconstructing an unknown bounded function defined on a domain from noiseless or noisy samples of at points . We measure the reconstruction error in a norm for some given probability measure . Given a linear space with , we study in general terms the weighted least-squares approximations from the spaces based on independent random samples. It is well known that least-squares approximations can be inaccurate and unstable when is too close to , even in the noiseless case. Recent results from [6, 7] have shown the interest of using weighted least squares for reducing the number of samples that is needed to achieve an accuracy comparable to that of best approximation in , compared to standard least squares as studied in [4]. The contribution of the present paper is twofold. From the theoretical perspective, we establish results in expectation and in probability for weighted least squares in general approximation spaces . These results show that for an optimal choice of sampling measure and weight , which depends on the space and on the measure , stability and optimal accuracy are achieved under the mild condition that scales linearly with up to an additional logarithmic factor. In contrast to [4], the present analysis covers cases where the function and its approximants from are unbounded, which might occur for instance in the relevant case where and is the Gaussian measure. From the numerical perspective, we propose a sampling method which allows one to generate independent and identically distributed samples from the optimal measure . This method becomes of interest in the multivariate setting where is generally not of tensor product type. We illustrate this for particular examples of approximation spaces of polynomial type, where the domain is allowed to be unbounded and high or even infinite dimensional, motivated by certain applications to parametric and stochastic PDEs.
DOI: 10.5802/smai-jcm.24
Mots-clés : multivariate approximation, weighted least squares, error analysis, convergence rates, random matrices, conditional sampling, polynomial approximation.
Albert Cohen 1; Giovanni Migliorati 1
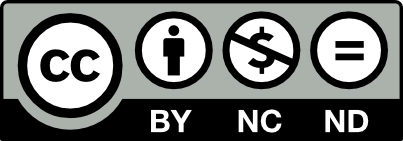
@article{SMAI-JCM_2017__3__181_0, author = {Albert Cohen and Giovanni Migliorati}, title = {Optimal weighted least-squares methods}, journal = {The SMAI Journal of computational mathematics}, pages = {181--203}, publisher = {Soci\'et\'e de Math\'ematiques Appliqu\'ees et Industrielles}, volume = {3}, year = {2017}, doi = {10.5802/smai-jcm.24}, zbl = {1416.62177}, mrnumber = {3716755}, language = {en}, url = {https://smai-jcm.centre-mersenne.org/articles/10.5802/smai-jcm.24/} }
TY - JOUR AU - Albert Cohen AU - Giovanni Migliorati TI - Optimal weighted least-squares methods JO - The SMAI Journal of computational mathematics PY - 2017 SP - 181 EP - 203 VL - 3 PB - Société de Mathématiques Appliquées et Industrielles UR - https://smai-jcm.centre-mersenne.org/articles/10.5802/smai-jcm.24/ DO - 10.5802/smai-jcm.24 LA - en ID - SMAI-JCM_2017__3__181_0 ER -
%0 Journal Article %A Albert Cohen %A Giovanni Migliorati %T Optimal weighted least-squares methods %J The SMAI Journal of computational mathematics %D 2017 %P 181-203 %V 3 %I Société de Mathématiques Appliquées et Industrielles %U https://smai-jcm.centre-mersenne.org/articles/10.5802/smai-jcm.24/ %R 10.5802/smai-jcm.24 %G en %F SMAI-JCM_2017__3__181_0
Albert Cohen; Giovanni Migliorati. Optimal weighted least-squares methods. The SMAI Journal of computational mathematics, Volume 3 (2017), pp. 181-203. doi : 10.5802/smai-jcm.24. https://smai-jcm.centre-mersenne.org/articles/10.5802/smai-jcm.24/
[1] A generalized sampling theorem for stable reconstructions in arbitrary bases, J. Fourier Anal. Appl., Volume 18 (2012), pp. 685-716 | DOI | MR | Zbl
[2] Sampling and reconstruction of solutions to the Helmholtz equation, Sampl. Theory Signal Image Process., Volume 13 (2014), pp. 67-89 | MR | Zbl
[3] Discrete least squares polynomial approximation with random evaluations - application to parametric and stochastic elliptic PDEs, M2AN, Volume 49 (2015), pp. 815-837 | DOI | MR | Zbl
[4] On the stability and accuracy of least squares approximations, Found. Comput. Math., Volume 13 (2013), pp. 819-834 | DOI | MR | Zbl
[5] Non-Uniform Random Variate Generation, Springer, 1985
[6] Coherence motivated sampling and convergence analysis of least squares polynomial Chaos regression, Comput. Methods Appl. Mech. Engrg., Volume 290 (2015), pp. 73-97 | DOI | MR | Zbl
[7] A Christoffel function weighted least squares algorithm for collocation approximations, Math. Comp., Volume 86 (2017), pp. 1913-1947 | MR | Zbl
[8] Szegö’s extremum problem on the unit circle, Annals of Mathematics,, Volume 134 (1991), pp. 433-453 | DOI | Zbl
[9] Multivariate Markov-type and Nikolskii-type inequalities for polynomials associated with downward closed multi-index sets, J. Approx. Theory, Volume 189 (2015), pp. 137-159 | DOI | MR | Zbl
[10] Convergence estimates in probability and in expectation for discrete least squares with noisy evaluations at random points, J. Multivar. Analysis, Volume 142 (2015), pp. 167-182 | DOI | MR | Zbl
[11] Analysis of discrete projection on polynomial spaces with random evaluations, Found. Comput. Math., Volume 14 (2014), pp. 419-456 | MR | Zbl
[12] Géza Freud, orthogonal polynomials and Christoffel Functions. A case study, J. Approx. theory, Volume 48 (1986), pp. 3-167 | DOI | Zbl
[13] Logarithmic Potentials with External Fields, Springer, 1997 | Zbl
[14] User friendly tail bounds for sums of random matrices, Found. Comput. Math., Volume 12 (2012), pp. 389-434 | DOI | MR | Zbl
Cited by Sources: